STORY #2
A Robotic Auditory Sense
That Detects Crises
in Shouts and Screams
Takahiro Fukumori, Ph.D.
Lecturer, College of Information Science and Engineering
An AI-incorporated system
distinguishing shouts and screams
that communicate crises has been developed
Sounds and voices are extremely important means of perceiving the world around us and our communication. Speech recognition and related technologies (such as those used to understand spoken words; gender and age; discernment of emotions like joy, anger, sorrow, and pleasure from speech; and determine the location and source of sounds based on its loudness and direction) have been evolving at an unprecedented pace in recent years.
Takahiro Fukumori, a lecturer at the College of Information Science and Engineering, is one such researcher trying to break new ground in the field of speech and environmental sound recognition. Fukumori is attempting to detect danger as conveyed in vocal sounds. Focusing his attention on shouts and screams as one of the elements indicating a critical situation, he aims to develop a “robotic auditory sense” that recognizes human shouts and screams and automatically detects whether or not a crisis is occurring. In recent studies, he has succeeded in developing a system using deep learning to distinguish calm voices from shouts and screams that signal danger or physical threat.
“Unlike typical voice recognition, what makes the determination of a critical situation based on shouts and screams difficult is that one never knows where and how a danger will present itself. To detect shouts or screams with a high level of accuracy, we must consider that they may be vocalized in noisy places filled with diverse sounds and noises, or at a distance from the microphone,” explains Fukumori.
According to Fukumori, MFCCs (Mel-frequency cepstral coefficients) are often used in conventional speech recognition to extract features important for such recognition. People produce various sounds by vibrating the vocal cords at the throat and allowing sound waves to pass through the throat, mouth, and other vocal tracts. MFCCs are designed to represent characteristics related to the vocal tract in the cepstrum domain. It features coarse sampling in the high-frequency bands and fine sampling in the low-frequency bands to match human auditory characteristics. “However, we have found that the movement of the vocal cords and vocal tract significantly differs in calm vocalization from that in shouts and screams,” says Fukumori. In other words, when people shout, their vocal cords and the vocal organs tend to vibrate more strongly, and the duration spent on pronouncing the trailing end of words tends to become longer than during calm speech. Therefore, MFCCs are not adequately able to capture the characteristics of shouts and screams.
Fukumori, who had been contemplating a solution to this problem based on prior research, observed the fact that energy in the harmonic component of a shout or a scream is stronger than that of calm vocalization. To detect a shout or a scream, he realized, it would also be effective to capture the characteristics of the spectrum (frequency) region of the speech and using both the spectral and cepstrum regions would improve detection accuracy.
First, he recorded voices of both men and women in calm conditions and shouts or screams suggestive of physical dangers. In total, he collected approximately 1,000 such samples. He then extracted both spectrogram and cepstrogram characteristics from each voice sample and trained a program using deep learning to build a model that separates calm voices from shouts and screams.
“Using this model, we conducted evaluation experiments and confirmed that it can detect shouts about 94.1% of the time on average, and about 80% of the time even under extremely noisy environments,” says Fukumori. They demonstrated that their model could identify shouts with higher accuracy than the conventional method using MFCCs and that its advantage became more apparent the noisier the environment became.
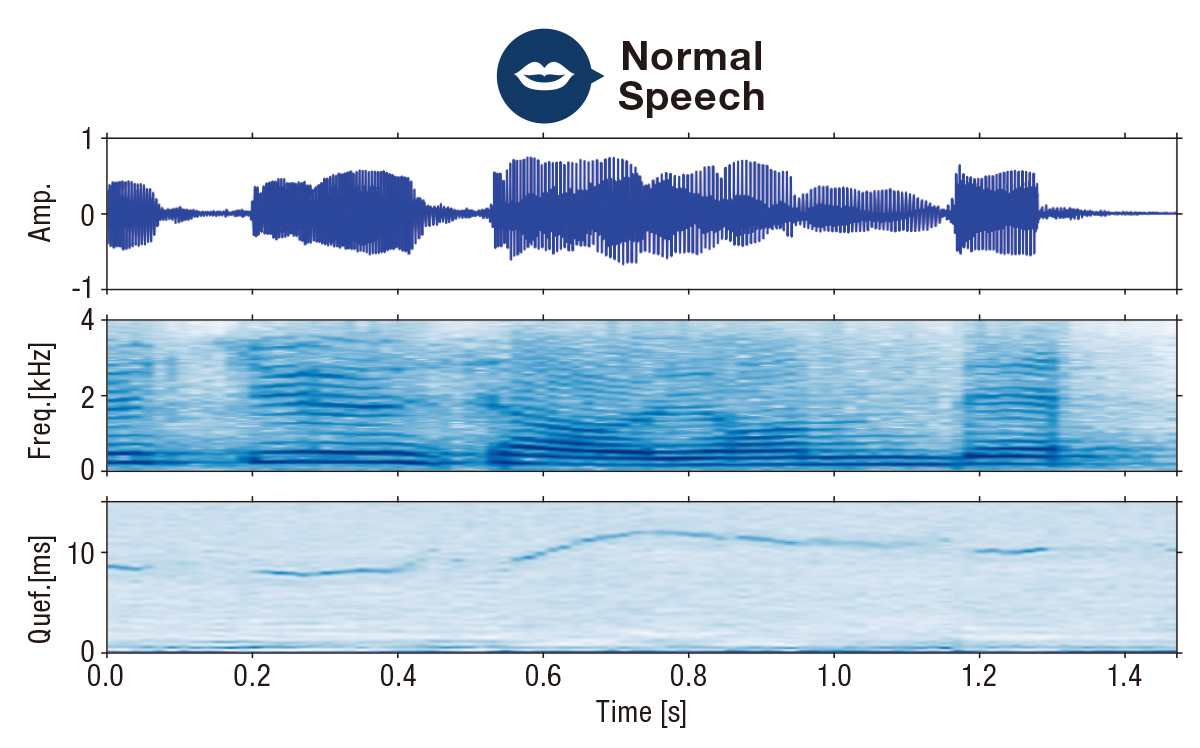
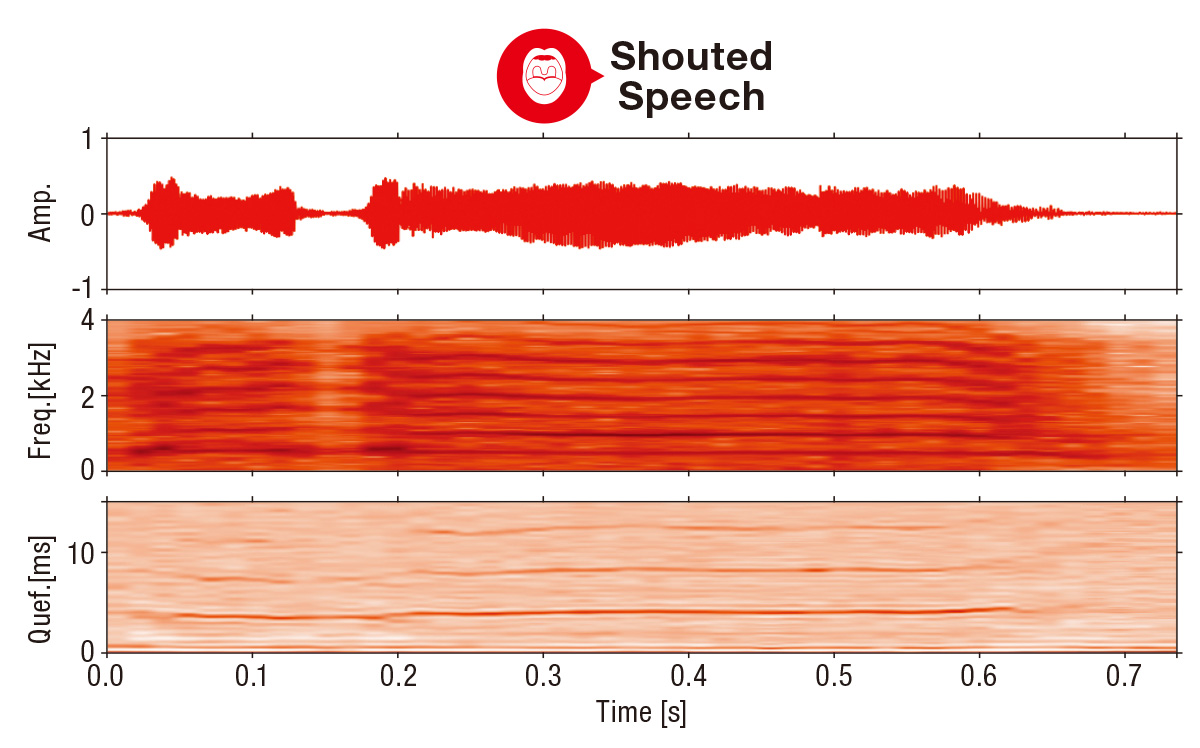
Results of analysis of calm voice (top) and shout (bottom).
The upper part of each diagram represents a waveform showing the time course of the sound intensity. The middle and lower parts represent the spectrograms and cepstrograms, respectively, used to differentiate a scream.
The same speaker utters the same word in each voice sample but with different characteristics. Thus, the key is to use a large number of audio samples to identify these differences through deep learning.
“While the penetration of AI technology has led to remarkable advances in voice recognition technology, the development of technology for robotics to detect crises through auditory means has lagged behind somewhat,” says Fukumori. One reason for this lag lies in the difficulty in collecting voice samples required for deep learning. What made Fukumori’s research successful was also due to his perseverance in collecting high-quality samples.
He is currently developing a system to estimate the intensity of the shouts and screams to determine how “scream-esque” they are. The first step is to enhance the voice samples further to include cries of delight and ecstasy in addition to screams and shouts and quantify the intensity of these sounds. Then he plans to have multiple people listen to shouts, screams, and yells and rate them on a scale of how “scream-esque” they were, and then use deep learning to build an estimation model based on this data.
“In addition to the intensity of the shouts and screams, I am also aiming to develop a system that could differentiate whether the shouts and screams are in reality communicating a crisis situation, or whether they are in fact positive vocal expressions like cheers and laughter,” says Fukumori. In the future, he hopes his research will lead to the development of a “crisis detection app” that could be integrated into smartphones and used in crime prevention in our daily lives.
Currently, cameras that use videos and image-based information to detect crimes and accidents have come into wide use. As if following suit, in recent years, “acoustic monitoring systems,” which use sound picked up by microphones to detect abnormal conditions, have been garnering attention. Speaking of the potential of robotic hearing, Fukumori says, “Robotic hearing compensates for the deficiencies of video and image information and is extremely effective in enhancing capabilities in surveillance and danger detection. Robots that can detect critical situations through hearing will be able to instantly detect violent incidents and major accidents so they can protect the public. I want to contribute to the advent of such an era.” We sincerely hope Fukumori’s research continues to grow and thrive.
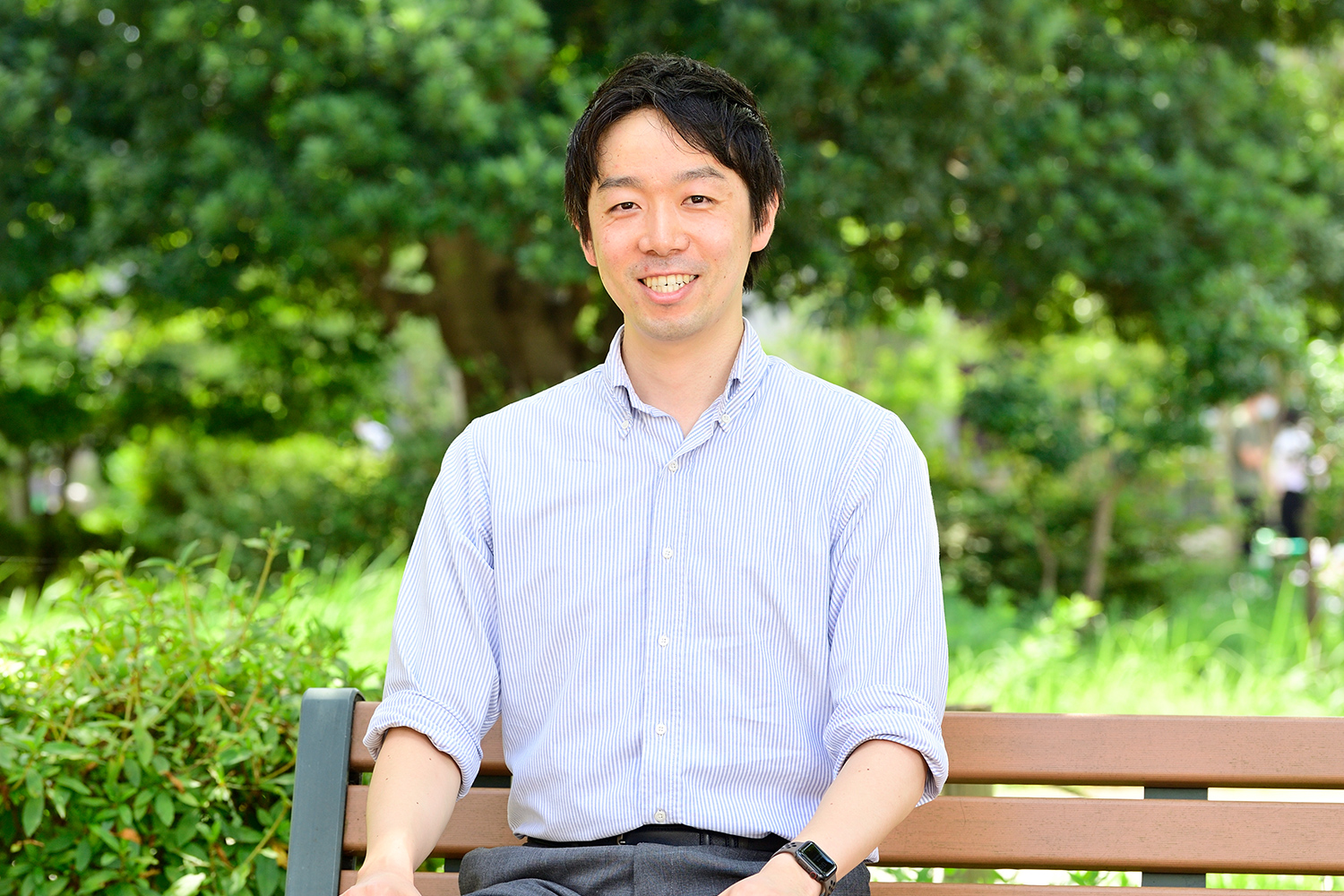
- Takahiro Fukumori, Ph.D.
- Lecturer, College of Information Science and Engineering
- Specialty: Perceptual information processing
- Research Themes: Estimation of speech state (e.g. shouting detection, speech intelligibility prediction, emotion recognition); Recognition and understanding of environmental sounds of everyday lives